Enhancing AI with Image Annotation Machine Learning
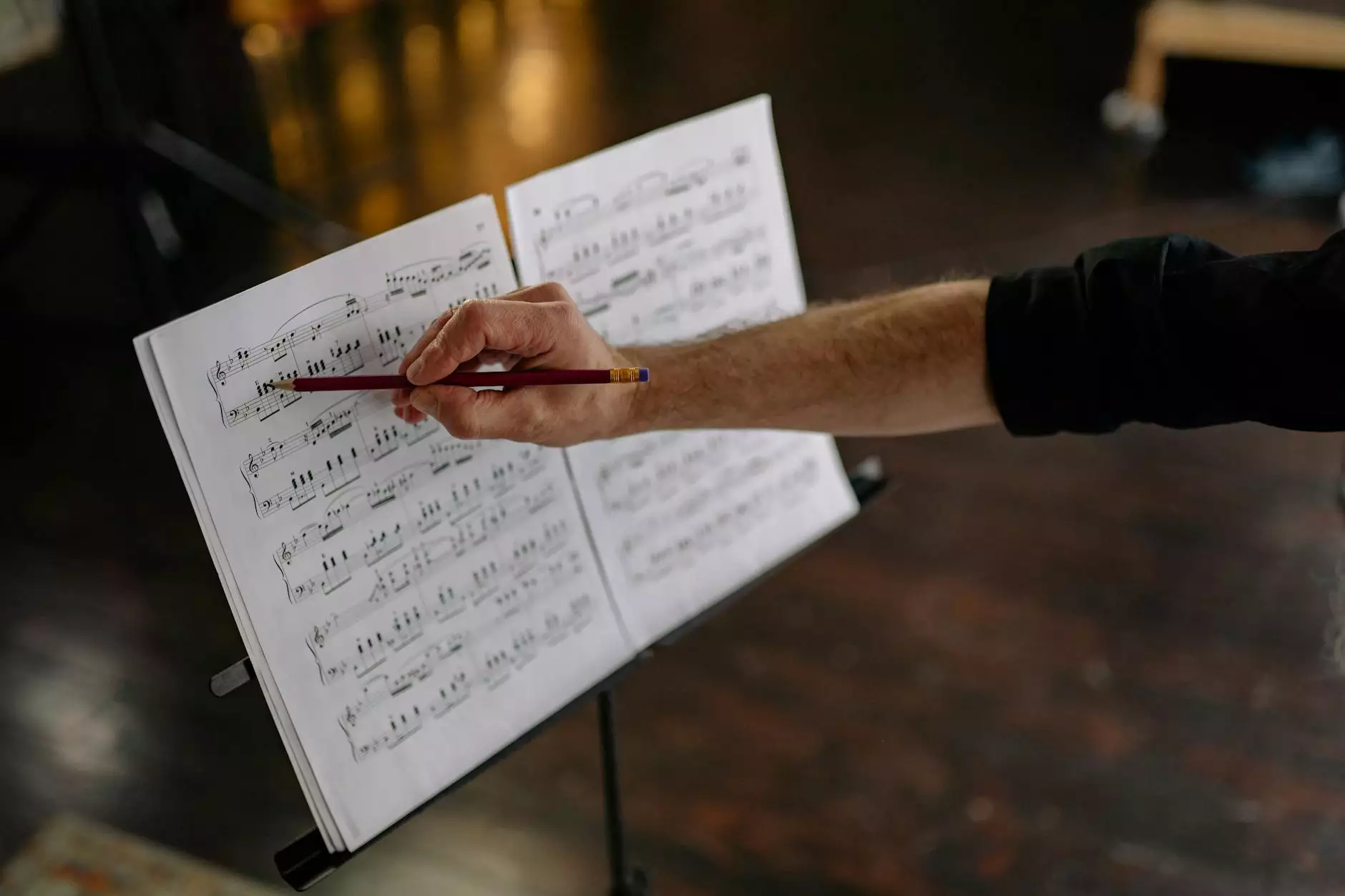
In the rapidly evolving world of technology, image annotation machine learning plays a crucial role in enhancing artificial intelligence capabilities. This process involves the use of various techniques to label and categorize visual data effectively, which is essential for training machine learning models. In this comprehensive guide, we will delve into the intricacies of image annotation, discuss its significance, and explore how platforms like Keylabs AI can streamline this process.
Understanding Image Annotation
At its core, image annotation is the process of adding labels and metadata to images, allowing machines to identify and understand the content within those images. This is particularly important in fields such as computer vision, where systems need to recognize objects, people, and scenes to function effectively.
What is Image Annotation Machine Learning?
Image annotation machine learning refers to the specialized application of machine learning techniques to automate the process of annotating images. By leveraging algorithms and pre-labeled data, machine learning models can learn to identify patterns and relationships within the data. This results in a more efficient annotation process, ultimately speeding up the training of AI systems.
Importance of Image Annotation in AI Development
The significance of image annotation cannot be overstated. Here are several reasons why it is foundational to the development of robust AI systems:
- Data Quality: Quality annotated data is critical for training machine learning models that deliver accurate predictions and classifications.
- Improved Performance: Models that are trained on well-annotated datasets tend to perform better in real-world applications, leading to enhanced reliability.
- Scalability: Automated image annotation through machine learning allows companies to scale their data annotation processes efficiently, handling vast amounts of data quickly.
- Cost-Effectiveness: By reducing the manual efforts involved in data annotation, businesses can significantly cut down on labor costs associated with model training.
Key Techniques in Image Annotation
Several techniques can be employed in the process of image annotation. Below are some of the most prevalent methods:
Bounding Box Annotation
Bounding box annotation is a fundamental technique where a rectangular box is drawn around the object of interest in the image. This method is widely used in applications such as object detection and tracking.
Semantic Segmentation
In semantic segmentation, every pixel in the image is classified into predefined categories. This allows for more granular understanding, enabling AI to recognize intricate details in images.
Instance Segmentation
Similar to semantic segmentation, instance segmentation separates different objects within an image, allowing the model to distinguish between individual instances of a single class. This is especially relevant in scenarios where overlapping objects may present challenges.
Landmark Annotation
Landmark annotation involves marking critical points on an object within the image. This technique is particularly useful in facial recognition and medical imaging, allowing algorithms to pinpoint specific features efficiently.
Applications of Image Annotation
The applications of image annotation are vast and varied, and they can be found across multiple sectors:
Healthcare
In healthcare, image annotation is pivotal for medical imaging analysis. Automated systems can detect anomalies in X-rays, MRIs, and CT scans, leading to quicker diagnoses and improved patient outcomes.
Autonomous Vehicles
For self-driving cars, image annotation is crucial for identifying objects such as pedestrians, other vehicles, and road signs, ensuring safe navigation through complex environments.
Retail and E-commerce
In the retail sector, computer vision technologies utilize image annotation to enhance customer experience by enabling visual search capabilities, personalized recommendations, and inventory management.
Agriculture
Farmers are harnessing image annotation for precision agriculture, using drones equipped with computer vision to monitor crop health and yield, as well as detect pest infestations.
Challenges in Image Annotation
While image annotation can significantly enhance AI capabilities, it also presents numerous challenges:
- Labeling Consistency: Maintaining a high level of consistency across annotated data is crucial, as discrepancies can lead to subpar model performance.
- Sample Diversity: A lack of diverse samples can limit the generalizability of the model, necessitating a comprehensive and varied dataset for effective training.
- Time-Intensive Process: Manual annotation can be labor-intensive and time-consuming, often hindering project timelines.
How Keylabs AI Transform Data Annotation Processes
Keylabs AI offers innovative solutions tailored specifically to overcome the challenges associated with image annotation. Their powerful data annotation tools and data annotation platform enable businesses to leverage machine learning capabilities for effective data labeling.
Accelerated Annotation with Automation
By integrating advanced automation into the annotation process, Keylabs AI allows for faster data labeling while maintaining high accuracy. This automated approach significantly reduces the time required for preparing datasets.
Collaboration Features
Collaboration is key in any data-driven project. Keylabs AI’s platform encourages teamwork among data scientists, engineers, and manual annotators to ensure the accuracy of annotations through continuous feedback loops.
Scalability Solutions
No matter the scale of your project, Keylabs AI is equipped to handle high volumes of data. Their infrastructure is designed to grow with your needs, adapting to a wide range of project sizes without compromising performance.
Comprehensive Support and Training
Keylabs AI provides extensive support and training for clients, ensuring that all users are proficient in using the platform. This is crucial for organizations that want to maximize their investment in data annotation tools.
Conclusion
The landscape of image annotation machine learning is more important than ever as businesses look to harness the power of AI. By understanding the various techniques, applications, and challenges involved, organizations can make informed decisions about their data annotation strategies.
With platforms like Keylabs AI, companies can streamline their annotation processes while benefiting from automation and support. The future of AI relies heavily on annotated images, and investing in high-quality data annotation tools is a step towards unlocking the potential of machine learning.
In conclusion, embracing image annotation not only enhances AI capabilities but also positions businesses to thrive in a data-driven world. Start your journey towards superior AI performance today by exploring the innovative solutions offered by Keylabs AI.